Artificial Intelligence for Image-Based Plant and Microbial Phenotyping
Authors:
John Lagergren1,2* ([email protected]), Larry York1,2, Mirko Pavicic1,2, Hari Chhetri1,2, Daniel Jacobson1,2, Tomas Rush1, Wellington Muchero1,2, David Weston1, Dale Pelletier1, Mitchel Doktycz1, Gerald Tuskan1,2 (PI)
Institutions:
1Oak Ridge National Laboratory; 2Center for Bioenergy Innovation
Goals
The Center for Bioenergy Innovation (CBI) vision is to accelerate domestication of bioenergy- relevant, non-model plants and microbes to enable high-impact innovations along the bioenergy and bioproduct supply chain while focusing on sustainable aviation fuels (SAF). CBI has four overarching innovation targets: (1) develop sustainable, process-advantaged biomass feedstocks; (2) refine consolidated bioprocessing with cotreatment to create fermentation intermediates; (3) advance lignin valorization for biobased products and aviation fuel feedstocks; and (4) improve catalytic upgrading for SAF blendstocks certification.
Abstract
There is a growing need to develop sustainable perennial crops and beneficial microbes that thrive in suboptimal environments, are resilient to biotic and abiotic stresses, and aid conversion to advanced bioproducts (e.g., sustainable aviation fuel). These goals are facilitated by connecting gene functions to observable traits through high-throughput measurement of key plant and microbe characteristics. However, crucial bottlenecks include the availability of large, high-quality datasets and the burden of time-, cost-, and labor-intensive phenotyping. Deep learning has enabled fast and accurate image-based phenotyping but is similarly challenged by the lack of annotated image data.
This presentation discusses ongoing efforts to address these challenges through the development of deep learning methods (Lagergren et al. 2023) that (1) maximize predictive accuracy while minimizing the amount of annotated training data; (2) ensure biologically realistic morphological features (e.g., contiguity); (3) scale to high-throughput, population-scale datasets for genomic analysis; and (4) extend across kingdoms and species (e.g., Populus spp., Bacillus spp.) and image modalities (e.g., RGB, hyperspectral, confocal microscopy). This team also shows how the model outputs (e.g., segmentations) are used to extract multiple traits (e.g., area, perimeter) using opensource tools (Seethepalli et al. 2021) that are validated with real-world physical measurements and used in downstream scientific analyses like genome-wide association studies.
Finally, the team introduces preliminary results that demonstrate how typical narrow artificial intelligence (e.g., models trained on specific datasets for a specific purpose as described above) struggle to generalize outside of their training distribution (e.g., adapting to new species, plant characteristics), and how foundation models for computer vision have recently emerged as powerful tools for image-based phenotyping with the ability to perform multiple tasks without the need to fine-tune or train new models from scratch. These new research directions are given in the context of the Advanced Plant Phenotyping Laboratory, a state-of-the-art plant phenotyping facility at Oak Ridge National Laboratory, which produces multimodal image data for DOE mission–relevant bioenergy crops at a rate of up to one petabyte per year. Harnessing the power of artificial intelligence through intentional development for biological sciences will be imperative to achieve sustainable energy independence for the nation.
Image
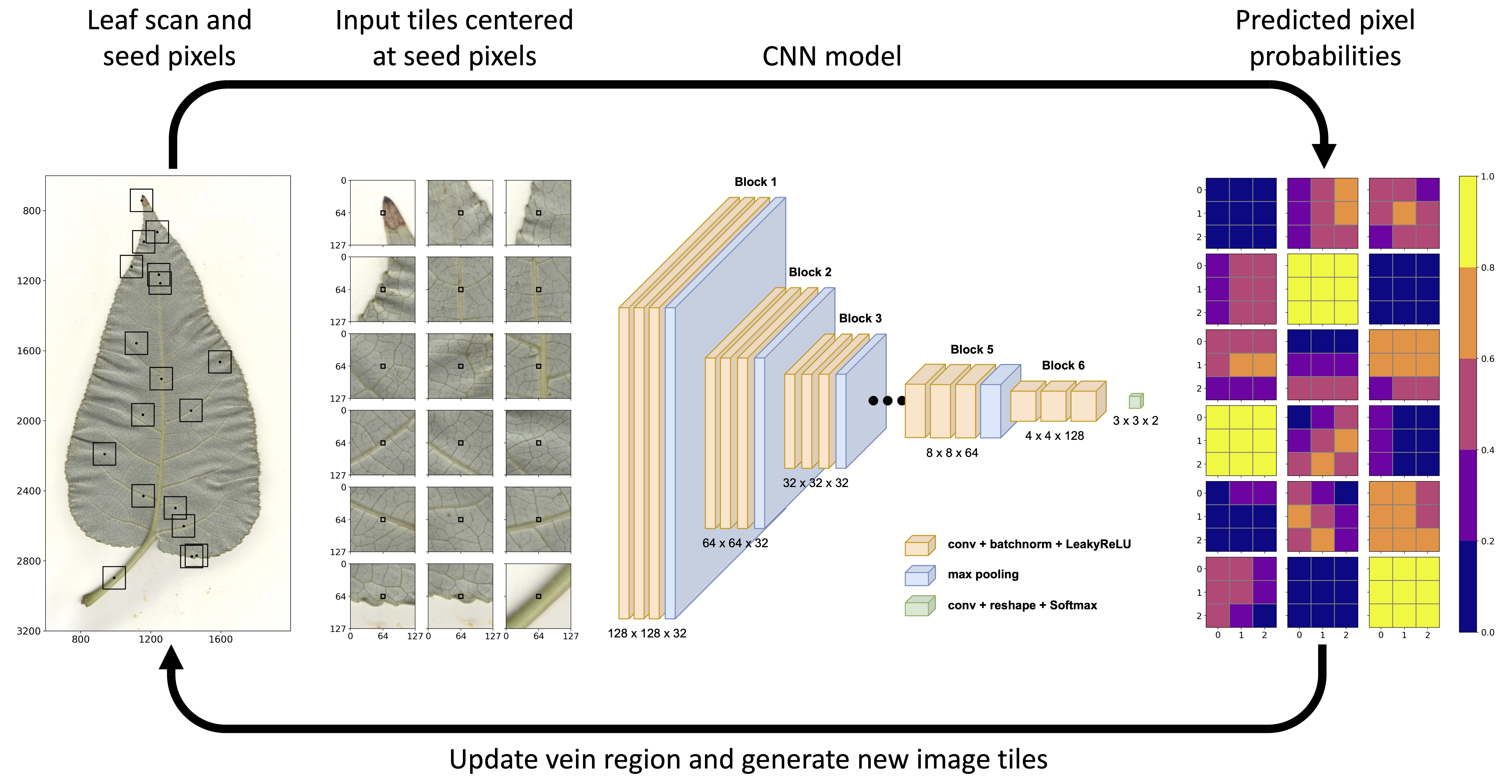
Leaf Tracing Algorithm. A neural network algorithm developed by John Lagergren that accelerates phenotyping by analyzing scanned images to identify key biologically relevant traits. [Reprinted from Lagergren, J., et al. 2023. "Few-Shot Learning Enables Population-Scale Analysis of Leaf Traits in Populus trichocarpa," Plant Phenomics 5. DOI:10.34133/plantphenomics.0072. Republished under Creative Commons license (CC BY 4.0)]
References
Lagergren, L., et al. 2023. “Few-Shot Learning Enables Population-Scale Analysis of Leaf Traits in Populus trichocarpa,” Plant Phenomics 5(75). DOI:10.34133/ plantphenomics.0072.
Seethepalli, A., et al. 2021. “RhizoVision Explorer: OpenSource Software for Root Image Analysis and Measurement Standardization,” AoB Plants 13(6). DOI:10.1093/aobpla/ plab056.
Funding Information
Funding was provided by the Center for Bioenergy Innovation (CBI) led by Oak Ridge National Laboratory. CBI is funded as a U.S. DOE Bioenergy Research Center supported by the BER program in the DOE Office of Science under FWP ERKP886. Oak Ridge National Laboratory is managed by UT-Battelle, LLC for DOE under contract DE-AC05-00OR22725.