Developing a Community Approach to Data Integration and Data Science in KBase
Authors:
Adam P. Arkin1* ([email protected], PI), Christopher S. Henry2, Robert Cottingham3, Shane Canon1, Paramvir S. Dehal1, Elisha Wood-Charlson1, Benjamin Allen3, Jason Baumohl1, Kathleen Beilsmith2, Joseph Bezouska2, David Dakota Blair4, John-Marc Chandonia1, Zachary Crockett3, Ellen G. Dow1, Meghan Drake3, Janaka N. Edirisinghe2, José P. Faria2, Jason Fillman1, Andrew Freiburger2, Tianhao Gu2, Prachi Gupta1, A. J. Ireland1, Marcin P. Joachimiak1, Sean Jungbluth1, Roy Kamimura1, Keith Keller1, Dileep Kishore3, Dan Klos2, Filipe Lui2, Mikaela McDevitt1, Christopher Neely1, Cody O’Donnell2, Erik Pearson1, Gavin Price1, Priya Ranjan3, William Riehl1, Boris Sadkhin2, Samuel Seaver2, Alan Seleman2, Gwyneth Terry1, Pamela Weisenhorn2, Sijie Xiang1, Ziming Yang4, Shinjae Yoo4, Qizhi Zhang2
Institutions:
1Lawrence Berkeley National Laboratory; 2Argonne National Laboratory; 3Oak Ridge National Laboratory; 4Brookhaven National Laboratory
URLs:
Goals
The DOE Systems Biology Knowledgebase (KBase) is a knowledge creation and discovery environment designed for biologists and bioinformaticians. KBase integrates a large variety of data and analysis tools, from DOE and other public services, into a user-friendly platform that leverages scalable computing infrastructure to perform sophisticated systems biology analyses. KBase is a publicly available and developer extensible platform, enabling scientists to analyze their own data alongside public and collaborator data, then share their findings across the system and ultimately publish reproducible analyses.
Abstract
KBase aims to empower its users to predict, control, and design the behavior of biological systems from subcellular to ecosystem processes. A critical capability for such research is the ability to find and integrate relevant data from the larger scientific community that can be used to strengthen and test the generality of user analyses, and to help identify gaps in both personal and collective knowledge that reduce the effectiveness of such analyses. To address the integration problem, this research group is leading two central efforts.
First, the group is working with partners at the DOE Joint Genome Institute (JGI), National Microbiome Data Collaborative (NMDC), and Environmental System Science Data Infrastructure for a Virtual Ecosystem (ESS-DIVE), among others, to develop a Data Transfer Service that streamlines finding and transporting data easily among, initially, BER platforms, while ensuring that provenance and ownership are tracked and credited. Researchers are developing an integrated system for scalable inference generation from user data, comprised of a central data model (CDM) containing the knowledge representation and data organization schema for the team’s system; a relation engine (RE) that powers the population of the CDM with public reference data; and a knowledge engine (KE) that interfaces with the RE to create a wide range of inferences for data entities within the CDM and for user data. Within KBase, these three elements (CDM, RE, and KE) work together to ensure data from diverse sources are linked by common concepts and thereby become comparable for analytical purposes.
The CDM is iteratively designed to represent biological, physical, and experimental relationships among data that are brought together from various resources and instantiated during intake into KBase. It will also enable queries supported by artificial intelligence (AI) to find and organize data relevant to a user’s question suitable for downstream analysis. This group intends for the CDM to serve projects beyond KBase and are assembling community members to aid in its design, testing, and iterative revision. The RE maps user data to the CDM and enables the creation, maintenance, and query of relationships within the CDM. The KE provides predicted and inferred relationships among data referenced by the CDM and creates facilities for data-driven search that enhance relevant data retrieval. The relationships include calculated similarity of genomes or genes, and predictions, such as phenotype and environmental distribution. The data-driven searches include sequenced or functional abundance profile-based queries that might return similar genomes or metagenomes to the user. The KE will also exploit new innovations in large language models and their interface to systems like the CDM to create AI-based assistants that enable users to employ natural language to state the problems they are trying to solve, and then navigate retrieving relevant data, organizing it alongside their own for analysis, and designing and executing the analyses with KBase tools.
This talk outlines the rationale and principles driving the development of the CDM and emphasize the importance of iterative community engagement throughout the process. In particular, this presentation outlines how it supports integration across resources and advancing biological data science within KBase. The goal is to ensure the CDM, and the tools it enables, will help lower the bar to data integration across BER, expand the types of science questions researchers can ask, and advance the field of data science to better handle the complexity in and among biological datasets this team’s scientists and the broader community are creating. This group will motivate this vision with examples drawn from causal microbial ecology that interfaces with a number of the goals of collaborating DOE programs.
Image
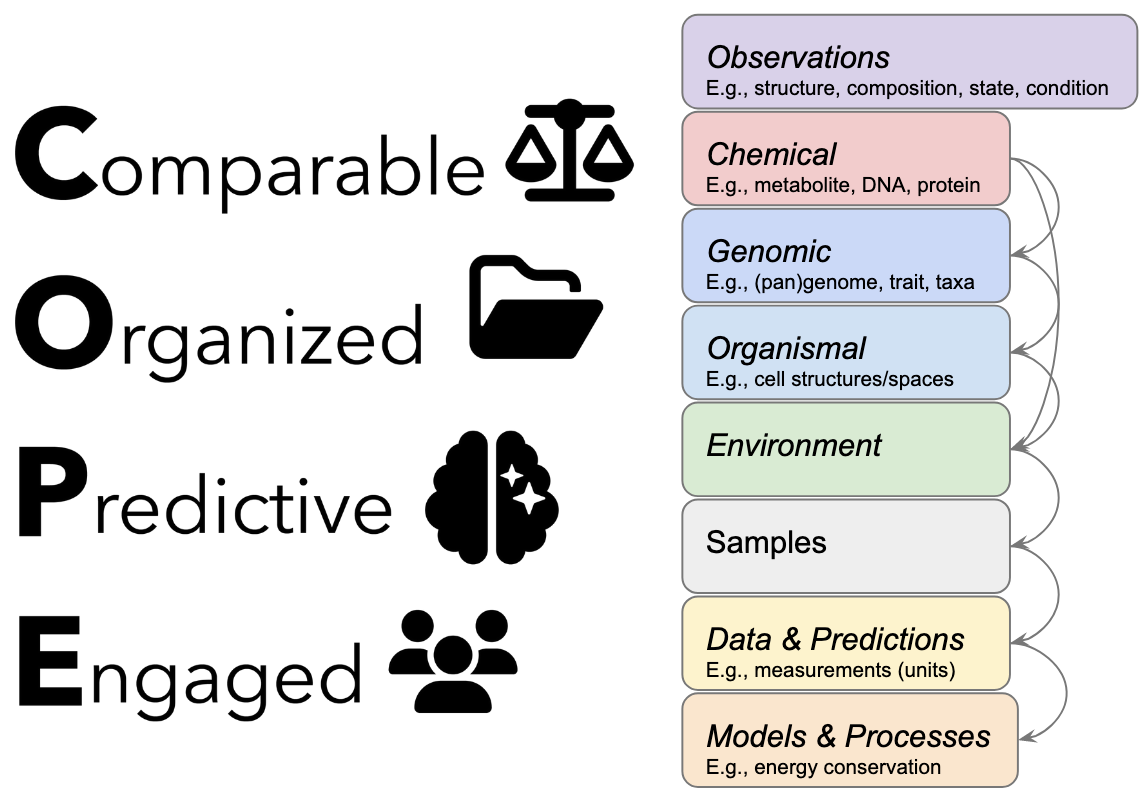
COPE. Going beyond findable, accessible, interoperable, reusable data requires a community effort to make data comparable and organized, and to increase its predictive potential by engaging in feedback and validation. [Courtesy DOE Systems Biology Knowledgebase]