Extracting Functional Traits from Large Volumes of Field Phenomics Data
Authors:
Emmanuel Miguel Gonzalez1* ([email protected]), Ariyan Zarei1, Jeffrey Demieville1, Nimet Beyza Bozdag1, Seungho Lee1, Sarthak Bawal1, Jordan Edward Pettiford1, Yuguo Xiao2, Indrajit Kumar2, Maxwell Braud2, Eric Lyons1, Duke Pauli1, and Andrea Eveland2
Institutions:
1University of Arizona; and 2Donald Danforth Plant Science Center
URLs:
- https://github.com/phytooracle
- https://datacommons.cyverse.org/browse/iplant/home/shared/phytooracle
Goals
- Use the University of Arizona’s Field Scanner to collect high spatial and temporal resolution field phenomics data on 430 ethyl methanesulfonate (EMS)-mutagenized families in the BTx623 background under well-watered and water limited conditions.
- Develop software and machine learning (ML) models to extract fine-scale phenotypic trait data at individual plant and organ levels from field phenomics data.
- Leverage fine-scale phenotypic trait data to study genotype-phenotype associations in response to drought to facilitate discovery of genes and their functions.
Abstract
Studying dynamic plant responses to environmental conditions has historically been difficult due to the low throughput and long-term cost of longitudinal data collection in the field setting (Reynolds et al. 2019). Recent technological advances have resulted in small, low-cost, and high-resolution sensors that can be used to rapidly collect phenotypic trait data at regular time intervals in field or greenhouse settings (Li et al. 2020; Sooriyapathirana et al. 2021). Today, high spatial and temporal resolution field phenomics data is being collected to extract information on dynamic plant responses to biotic and abiotic stress under real world field conditions. When phenotypic trait data from multiple sensors are combined, a multidimensional understanding of plant morphology and physiology can facilitate the discovery of genes and their functions. The University of Arizona is home to the world’s largest outdoor plant phenotyping system, the Field Scanner that encompasses numerous sensors for collecting plant phenotypic trait data. The Field Scanner collects red-green-blue (RGB), photosystem II (PSII) chlorophyll fluorescence, and thermal images as well as 3D point clouds using laser scanners. The Field Scanner raw data is being processed using PhytoOracle (PO), a series of scalable, modular phenomic data processing pipelines (Gonzalez et al. 2022). The processed data generated by PO is enabling the extraction of increasingly fine-scale traits from various levels, from the field to plot and whole plant to individual organs. To extend phenotyping capabilities, novel machine learning (ML) algorithms are being developed to extract multidimensional phenomics datasets that can be mined for genotype-phenotype associations related to abiotic stress.
ML models that aim to segment plant point clouds can provide fine-scale phenotypic data on plant morphology at individual plant and organ levels (Figure 1). Various traditional shape descriptors can be extracted, including height, volume, and angle. Additionally, topological data analysis (TDA), a mathematical framework for studying the underlying connections of points and their properties, can be used to study shape nuances that may not be captured by traditional shape descriptors. Persistence diagrams and Euler characteristic curves are common TDA methods, which aim to capture topological signatures that summarize shape features from which shape nuances can be studied (Amézquita et al. 2022.; Amézquita 2020; Chazal and Michel 2021). A variety of traditional and TDA shape descriptors are being collected from 430 EMS-mutagenized sorghum families in the BTx623 background under well watered and water limited conditions across the life cycle of plants. These shape descriptors are being leveraged to identify genotype-phenotype associations related to drought stress to enable gene discovery. This work will identify induced variation in drought resilient traits for enhancing the productivity of bioenergy crops under drought conditions through fine-scale phenotyping. This information will drive the breeding and engineering of improved, climate-resilient varieties capable of maintaining productivity under limited resources.
Image
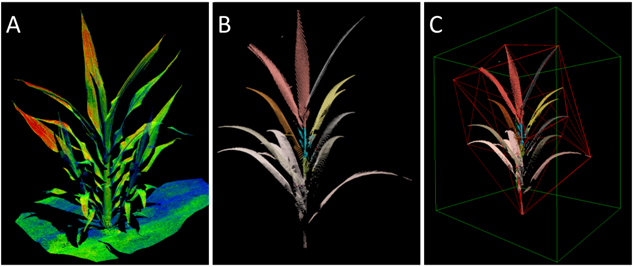
Figure 1. Sorghum individual plant phenotyping workflow. (A) Individual plants are isolated from raw field phenomics data. (B) Machine learning (ML) models segment soil from plant points and classify distinct leaves. (C) Various leaf and whole-plant phenotypes are extracted, including volume, topological data analysis (TDA) shape descriptors, and leaf descriptors.
References
Reynolds, D., et al. “What is Cost-Efficient Phenotyping? Optimizing Costs for Different Scenarios,” Plant Science 282, 14–22.
Li, B., et al. 2020. “Phenomics-Based GWAS Analysis Reveals the Genetic Architecture for Drought Resistance in Cotton.” Plant Biotechnology Journal 18, 2533–44.
Sooriyapathirana, S. D. S. S. et al. 2021. “Photosynthetic Phenomics of Field- and Greenhouse-Grown Amaranths vs. Sensory and Species Delimits. Plant Phenomics 2021, 1–13.
Gonzalez, E., et al. 2022. PhytoOracle Automation (Version 1.0.0). [https://github.com/phytooracle/automation]
Amézquita, E. J., et al. “Measuring Hidden Phenotype: Quantifying the Shape of Barley Seeds Using the Euler Characteristic Transform.” Silico Plants 4, diab033.
Amézquita, E. J., et al. 2020. “The shape of things to come: Topological data analysis and biology, from molecules to organisms.” Developmental Dynamics 249, 816–833.
Chazal, F., and B. Michel. 2021. “An Introduction to Topological Data Analysis: Fundamental and Practical Aspects for Data Scientists.” Frontiers in Artificial Intelligence 4.
Funding Information
This material is based upon work supported by the U.S. Department of Energy Biological and Environmental Research Award Number DE-SC0020401, the U.S. Department of Energy Advanced Research Projects Agency-Energy OPEN Award Number DE-AR0001101, and the National Science Foundation CyVerse Award Number DBI-1743442.